Home >  Courses > Trending Courses > Data Science Course
Data Science Training in
Hyderabad
Data Science is a multidisciplinary field that combines techniques from statistics, mathematics, computer science, and domain knowledge to extract insights and valuable information from large sets of data. It involves analyzing, interpreting, and visualizing data to solve complex problems and make data-driven decisions.
14 Modules
with Certifications
Certificate
After Completion
English
Language
A Data Scientist is a skilled professional who possesses a deep understanding of data analysis, machine learning algorithms, programming, and domain expertise. They have the ability to uncover patterns, trends, and correlations in data, enabling organizations to make informed decisions and gain a competitive edge. So, Join Version IT’s Data Scientist Training in Hyderabad program to become a Data Scientist.
Why Should you Enroll in a Data Science? Â
The demand for skilled Data Scientists has skyrocketed in recent years across various industries. Here are some compelling reasons why you should consider taking a Data Science course:
Lucrative Career Opportunities:Â Data Science professionals are in high demand, with companies across industries seeking their expertise to extract valuable insights from data. Pursuing a career in Data Science can lead to lucrative job opportunities and attractive salary packages.
Exciting and Challenging Work:Â Data Science involves solving complex problems and uncovering hidden patterns in data. If you have a passion for solving puzzles and enjoy intellectual challenges, Data Science offers a stimulating and fulfilling career path.
Continuous Learning and Growth:Â Data Science is a rapidly evolving field, with new techniques, algorithms, and tools emerging regularly. By taking a Data Science course, you can stay updated with the latest advancements and continue learning throughout your career.
Why is Version IT the best data science training institute in Hyderabad?
Version IT stands out as the premier Data Science training institute in Hyderabad for the following reasons:
Expert Faculty:Â We have a team of 15+ years of experienced and industry-expert instructors who bring their real-world experience to the classroom. They provide comprehensive guidance, mentorship, and practical insights to ensure you receive the best education in Data Science.
Comprehensive Curriculum: Our Data Science course in Hyderabad offers a comprehensive curriculum that covers all the essential concepts, tools, and techniques of Data Science. We focus on practical application through hands-on projects, enabling you to build a strong foundation and gain valuable experience.
Hands-on Approach:Â We believe in a hands-on learning approach where you actively work on real-world projects, analyze datasets, and implement algorithms. This practical experience helps you develop the necessary skills and prepares you for real-world challenges.
Who Should Do the Data Science Course?
The Data Science course is suitable for professionals and aspiring individuals from various backgrounds, including:
IT Professionals:Â Data Science allows IT professionals to upskill and enhance their career prospects. Those with a background in programming, software development, or data analysis can leverage their existing knowledge and transition into the field of Data Science.
Domain Experts:Â Professionals with domain expertise in fields such as finance, healthcare, marketing, or retail can leverage Data Science to gain valuable insights and optimize processes within their industries.
Graduates and Postgraduates:Â Fresh graduates and postgraduates from computer science, mathematics, statistics, or engineering can kickstart their careers in Data Science by acquiring the necessary skills and knowledge through a Data Science course.
What is the course Objective
The objective of our Data Science course is to equip you with the essential skills and knowledge required to become a successful Data Scientist. After completing the course, you will:
- Gain a solid understanding of fundamental Data Science concepts, techniques, and algorithms.
- Learn to analyze, interpret, and visualize data using various tools and programming languages.
- Acquire hands-on experience by working on real-world projects and datasets.
- Develop the ability to apply machine learning algorithms and statistical models to solve complex problems.
- Master the art of storytelling through data visualization and communicate insights effectively.
- Gain proficiency in using popular Data Science tools and libraries.
- Be well-prepared to enter the job market as a skilled Data Science professional.
Enroll in Version IT’s Data Science training in Hyderabad and embark on an exciting journey toward a successful career in the dynamic field of Data Science. Contact us today for one of the Recommended Data Science Institutes in Hyderabad to learn Data science.
Topics You will Learn
Introduction to DataScience
- What is data science?
How is data science different from BI and Reporting? - What is the difference between AI, Data Science ,Machine Learning ,Deep Le Deep Learning, and Job Landscape and Preparation Time?
- Who are data scientists?
- What skill sets are required?
- What is day to day job of Data Scientist
What kind of projects they workon? - End toEnd Data Sciene Project Life Cycle
Data Science roles, functions ,payacross domains, experience
Business Statistics
Datatypes
- Continuous variables
- Ordinal Variables
- Categorica lvariaes
Time Series - Miscellaneous
- Common Data Science Terminology
Descriptivestatistics
- Basicscon ceptsofprobability
- Frequentis versus Bayesian Probability
- Axioms of probability theory,
- Permutation and sand combinations
- Conditional and marginal probability
- Joint Probability
- Bayes Theorem
- Probability Mass Function and Probability Density Function Cumulative Mass Function and Cumulative Density Function
Centra lTendencies
- Mean
- Median
- Mode
- Spread
- Variance
- Standard Deviation
Effects on central tendencies after transformations
QuartileAnalysis
Implementation ofcentraltendenciesusingpython
Box Plotsforoutlieridentification
Drawing Boxplotsusingpython
Sampling
- Need forSampling?
- Different typesofSampling
- Simpler andomsampling
- Systematic sampling
- Stratified Sampling
- Implementation of sampling techniques using Python
Datadistributions
- Normal Distribution
- Binomial Distributin
- Binomial Approxi maed to Normal
- Implementation of distributions using python
Inferential statistics
- Why inferential statistics?
- Z score calculation
- Defining value and implementations using python
- Inferring from the sample population
- Sampling distribution of sample means
Hypothesis testing
- Confiden ceInterval
- Testing thehypothesis
- Type Ierror
- Type IIerror
- Null and alternate hypotheses
- Reject or acceptance criteria
Introductiontor
- APrimertoRprogramming
- WhatisR?SimilaritiestoOOPandSQL
- TypesofobjectsinR–lists,matrices,arrays,data.framesetc.
- Creatingnewvariablesorupdatingexistingvariables
- Ifstatementsandconditionalloops-For,whileetc.
- Stringmanipulations
- Subsettingdatafrommatricesanddata.frames
- Castingandmeltingdatatolongandwideformat. i. Mergingdatasets
Python for Data Science
- Understanding the reason of Python’s popularity
- Basics of Python :Operations , loops, functions, dictionaries
- Numpy–creating arrays, reading, writing, manipulation techniques
- Ground-up for Deep-Learning
Exploratory Data Analysis with Python
- Getting to understand structure of Matplotlib
- Configuring grid, ticks.
- text, colormap, markers ,widths with Matplotlib
- configuringaxes, grid,
- hist, scatterplots
- barcharts
- multipleplots
- h.3Dplots i. Correlationmatrixplotting
DataMungingwithPython
a.Introduction to pandas
b. Data loading with Pandas
c. Data types with python
d. Descriptive Statistics with Pandas
e. Quartile analysis with Pandas
f. Sort, Merge, join with Pandas
g. Indexing and Slicing with pandas
h. Pivottable, Aggregate and crosstab with pandas
i. Applyfunction for parallel processing with Python
j. Cleaning Data with python
k. Determining correlation
l. Handling missing values
Practical use cases of AI and best practices in AI
a. Business-problem analytical problem
b. Guidelines for development
Analytical Visualisati on withTableau
a. Why is it important for data analysts?
b. Tableauwor k bookwalkthrough
c. Instructions for your own workbooks
d.Demonstrate more workbooks.
Introduction to Artificial Intelligence
a. Dealing with Prediction Problems
b. Fore casting for industry
c. Optimise on in logistics
d. Segmentation customer analytics
e. supervised learning
f. Unsupervised Learning
g. Optimisation
h. Types of AI: Statistical Modelling, Machine Learning, and Deep
Learning, Optimisation, Natural Language Processing, and Computer Vision, Speech Processing, Robotics
ArtificialIntelligenceI-StatisticalModelling
- a. Linear Regression
 Assumptions - Model development and interpretation
- Sum of least squares
-  Model validation–
- tests to validate assumptions
- Multiple linear regression
- Disadvantages of linear models
- b. Logistic Regression
- Maximum likelihood estimation
- Â Model development and interpretation
 Confusion matrix-error - measurement ROC curve
 Measuring sensitivity and specificity
Advantages and disadvantages of the logistic regression models - c. Timeseries analysis: forecasting
1. Simple moving averages
2. Exponential smoothing
3. Time series decomposition
4. ARIMA - d. Model validation and deployment
- RMSE-RootMean squarederror
 - MAPE: Mean Average Percentage Error
- Confusion matrix and misclassification rate
Area under the curve (AUC), ROC curve
ArtificialIntelligenceII–MachineLearning
Supervised LearningÂ
1.Decisiontrees and Random Forest C5.0
2. Classification and regression trees (CART)
3. Process of tree building
4. Entropy and Gini Index
5. Problem of overfitting
6. Pruning a tree back
7. Trees for Prediction (Linear): Example
8. Tress for classification models—example
basedmodels?Association Rule Mining
1.Rulesgene ration from decision trees,
2. Apriori algorithm
3.Support,confidence and lift measures Support
Vector Machines
1.Support, confidence, and lift measures
RT Vector Machines Linear learning machines
2.SVM case for linearly separable data
3. Kernel space Vector Machines
Neural Networks
1. Motivation for Neural Networks
2. Perceptron and Single-Layer Neural Network
3. Back Propagation Algorithm
4. Feed Forward Neural Net
5. Sigmoid parameters
6. Weight initialization,
7. Decay of weights
8. Learning rate
9. Momentum
Ensemble Techniques
1. Bagging
2. Boosting
3. Stacking
10.Gradient Boosting Machines
b. Unsupervised Learning
Clustering Techniques
1. Hierarchical clustering
2. K-Means clustering
3. Distance measures
4. Applications of cluster analysis and customer segmentation
Collaborative
Filtering ,PCA
Artificial Intelligence III: Natural Language Processing
a. NLPI-Text Pre Processing
 Tokenization
 Stemming
 Lemmatization
b. NLPII-Text Modelling
  POS tagging
TFIDF and classificationÂ
Artificial Intelligence IV-Deep Learning
a. ReLU
b. Sigmoid, Depth vs. Width Tradeoffs
c. Convolutional Networks
d. Concept of filters
e. Sliding
f. Pooling and padding
g. Comparis on between DL and ML performances over the MNIST dataset
Big Data,Azurefor AI, and Data Science Applications
a. Big data analytics?
  Leverage big data platforms for data science.
b. Introducti ontoevolvingtoolsÂ
 Machine learning with Spark
c. Creation of R-Server Clusters
Let Your Certificates Speak
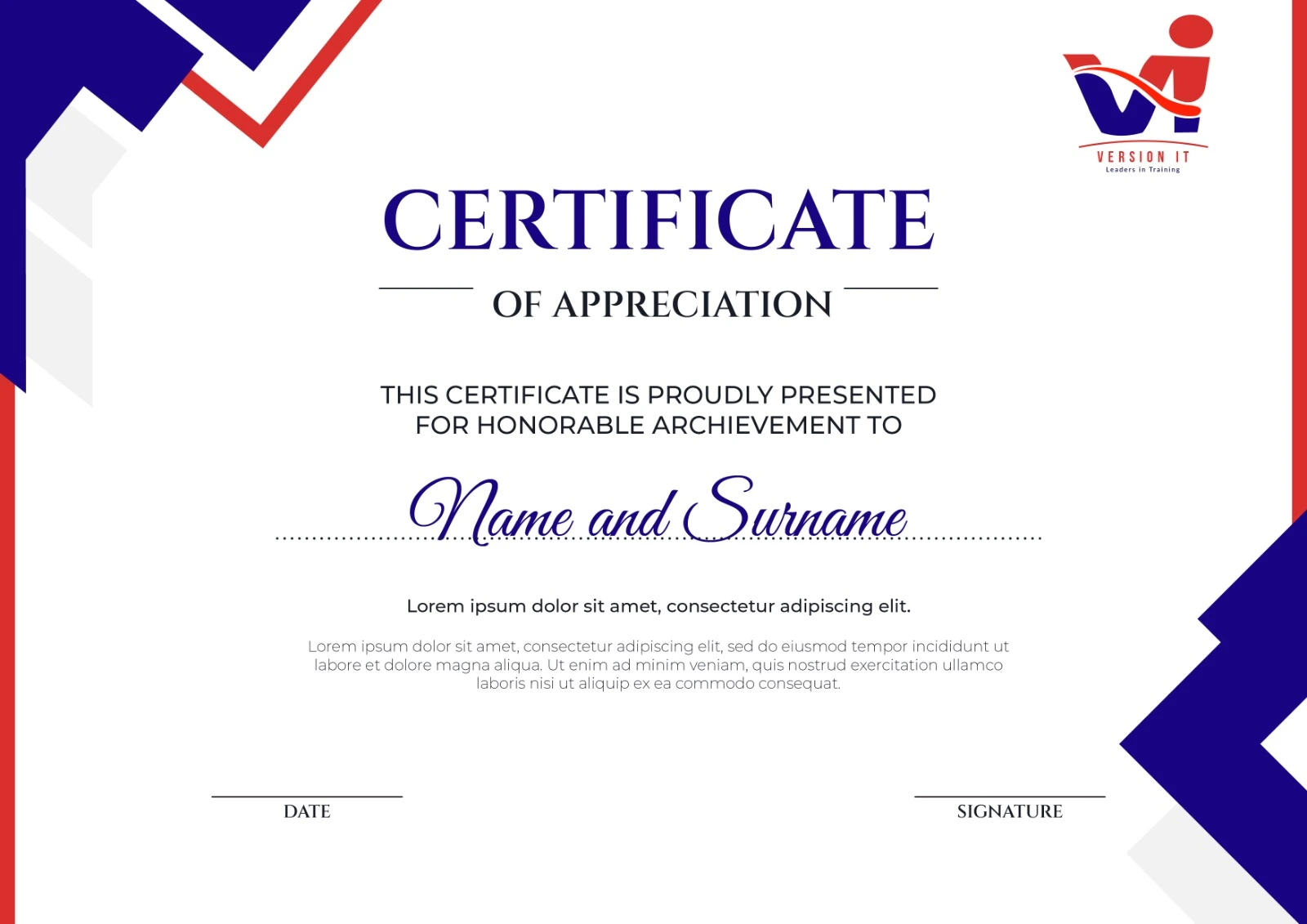
- Acquiring an extensive education in data science necessitates a blend of conceptual understanding, pragmatic abilities, and actual implementations.
- Certificates are globally recognized & they upgrade your programming profile.
- Certificates are generated after the completion of course.
All You Need to Start this Course
- Mathematics and Statistics: Learn the fundamentals of calculus, linear algebra, and statistics. Numerous algorithms in data science are built on top of this.
- Learning a programming language that is often used in data science is strongly advised.
Testimonials
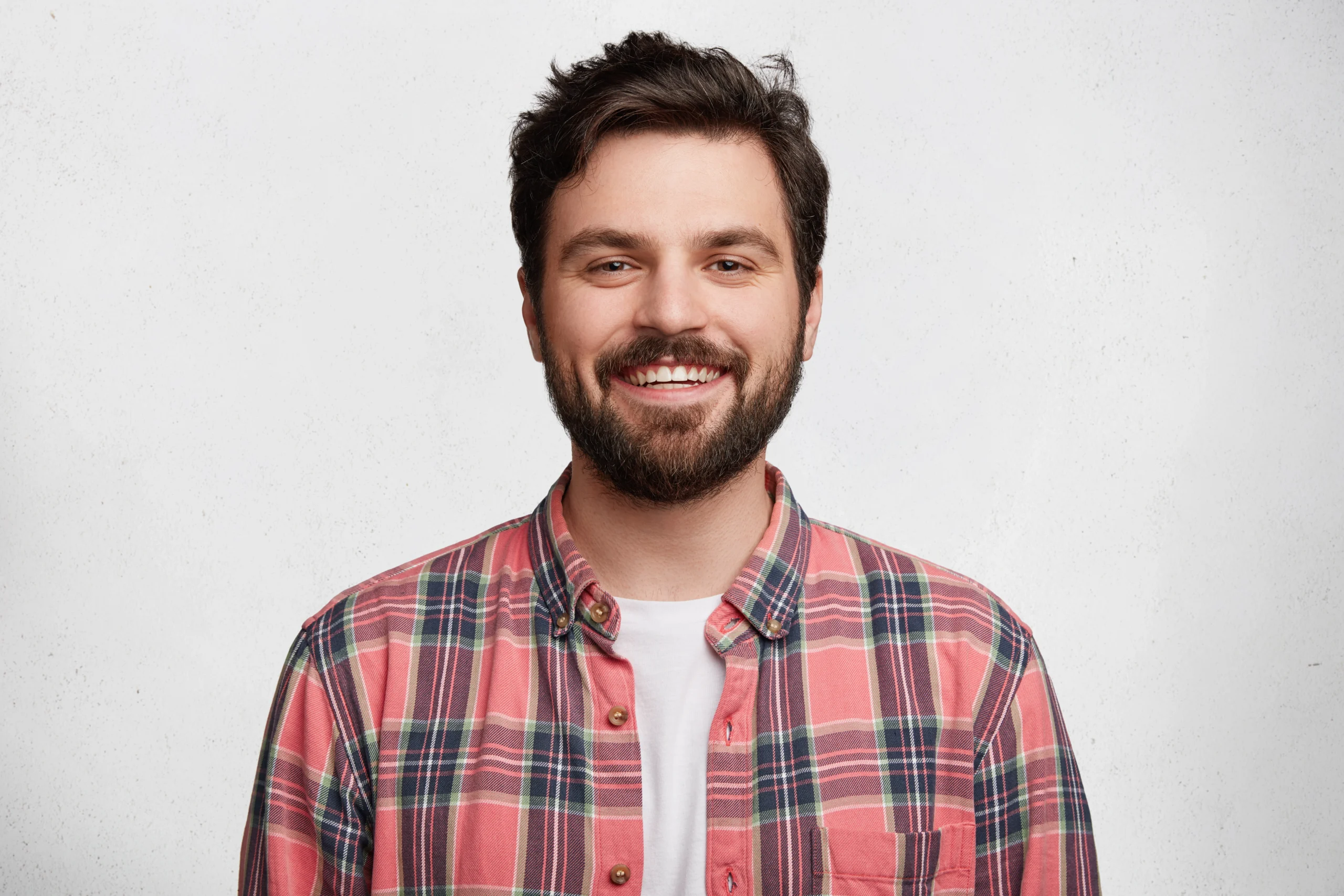
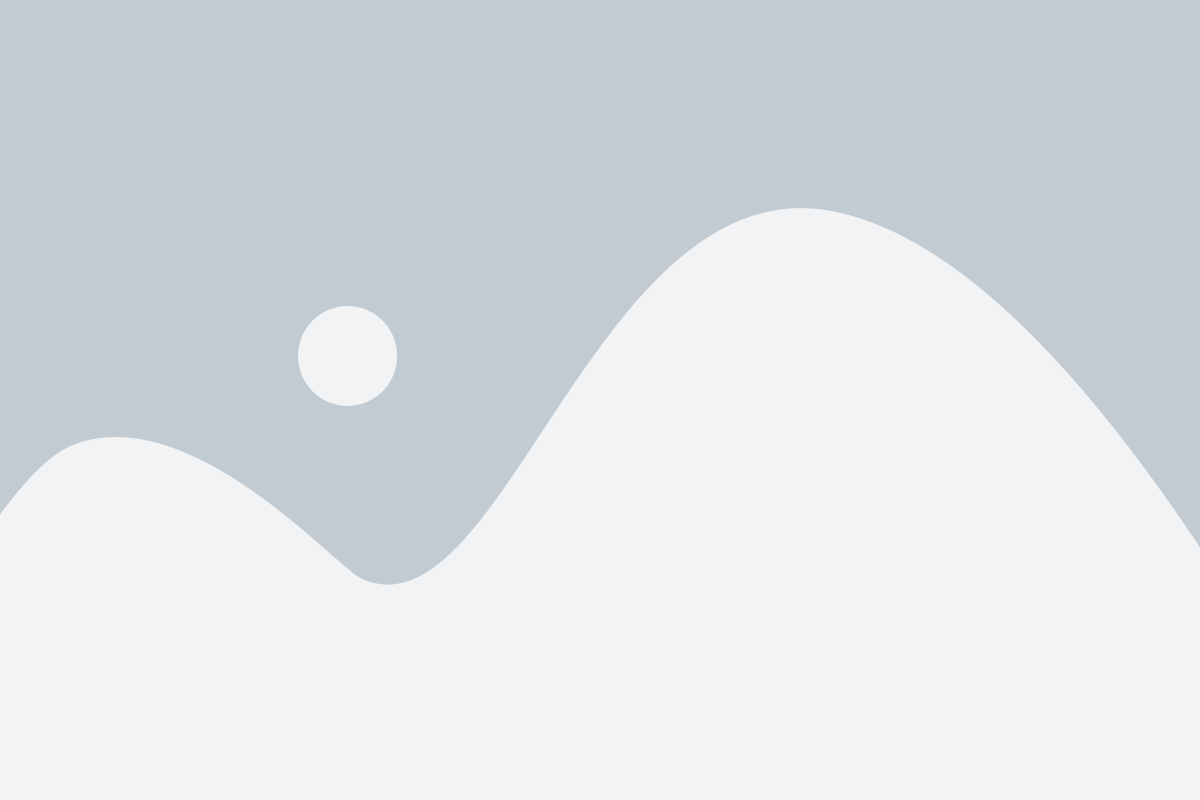
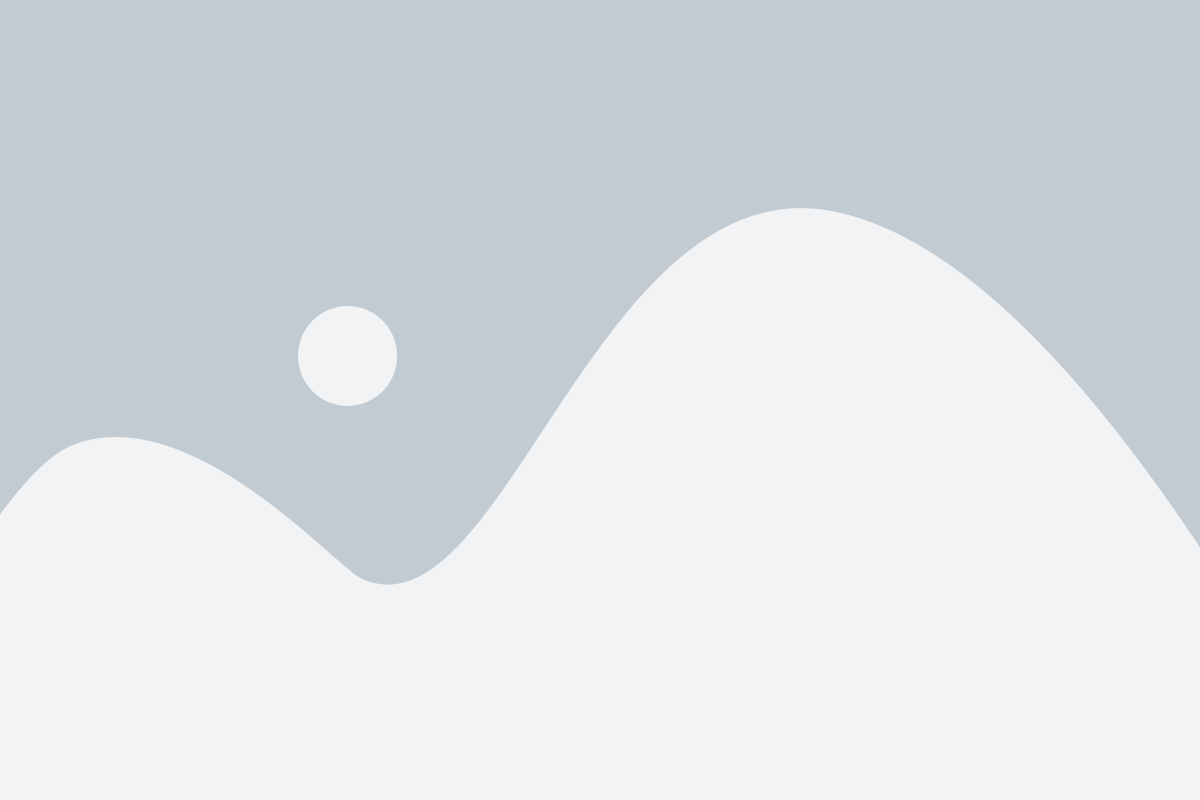
Still Having Doubts?
Data science is an interdisciplinary field that extracts knowledge and insights from both structured and unstructured data using scientific procedures, systems, algorithms, and methodologies..
Key competencies include domain-specific expertise, statistics, machine learning, data visualization, data cleaning, and programming languages (such as Python and R). Soft skills like good communication and critical thinking are equally crucial.
The problem formulation, data collecting, data cleaning and preprocessing, exploratory data analysis, feature engineering, model development, model evaluation, and deployment are standard steps in the data science pipeline. It's frequently depicted as a cycle since models may need to be adjusted in response to fresh information or shifting specifications.